Agentic AI in Business and Industry
- GSD Venture Studios
- 5 days ago
- 10 min read
By Gary Fowler
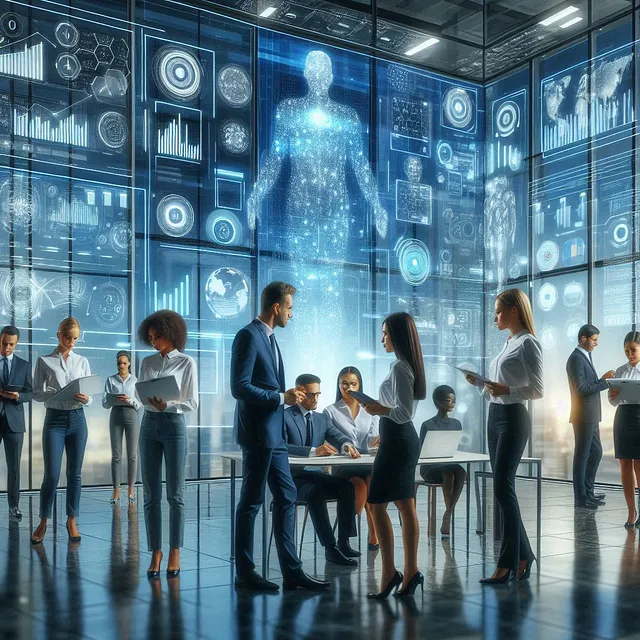
Introduction
Picture a world where software agents think on their own, learn from complex scenarios, and make decisions in real time — without needing constant human intervention. That’s the essence of “Agentic AI.”
In today’s rapidly shifting market landscape, we see companies striving to streamline their operations, reduce costs, and build up their innovation pipeline. Agentic AI is taking center stage in this transformation. Rather than just reacting to commands, these intelligent systems operate with a level of autonomy that can feel almost human, enabling businesses to push boundaries in efficiency, optimization, and collaboration.
Defining Agentic AI
Agentic AI refers to artificial intelligence systems capable of making decisions and taking actions independently. These AI agents can navigate uncertainties, adjust to new environments, and continuously optimize their behaviors based on incoming data. Let’s break down what makes them special.
Key Characteristics
Autonomy: Agentic AI isn’t waiting around for constant human instructions. It has the freedom to make decisions within specific constraints, learning from real-time data or historical patterns.
Adaptability: These AI systems evolve when presented with new challenges. If a fresh, unexpected variable appears, agentic AI can adapt its strategy on the fly.
Goal-Oriented: Agentic AI operates with specific objectives in mind, leveraging data analytics and predictive algorithms to move closer to its goals.
Distinguishing from Reactive AI
While reactive AI systems respond only to immediate stimuli — think of a spam filter identifying suspicious emails — agentic AI goes a step further. It not only reacts but also plans, envisions multiple scenarios, and takes proactive measures. Reactive AI is limited to direct inputs, whereas agentic AI employs context and history to make more autonomous decisions.
The Growing Role of Agentic AI
Evolution of AI in Business
AI has been transforming businesses for quite some time, starting with basic automation. We witnessed early AI in the form of rule-based expert systems, where input-output patterns were defined by rigid coding. Over the past few decades, with the rise of machine learning, we gained AI that can learn from examples instead of relying solely on pre-established rules. Now we’re entering an era where AI agents can act proactively. Rather than passively analyzing data, they dive in, make decisions, and adapt their strategies.
Industry Adoption Trends
Agentic AI isn’t a hypothetical. From supply chain optimization to advanced robotics, industries worldwide are racing to integrate intelligent agents. Leading companies in manufacturing, finance, healthcare, retail, and tech are at the forefront. As these AI systems become more affordable and easier to implement, even small and mid-sized businesses can embrace agentic AI to boost efficiency and gain a competitive edge.
Key Components of Agentic AI
Autonomy
Autonomy allows AI agents to operate under a set of guidelines but without constant oversight. They receive data, process it, and make real-time decisions. This autonomy is critical in fast-moving scenarios — like a high-frequency trading algorithm or a robotic arm on an assembly line — that can’t afford to wait for a manager to step in.
Adaptability
Agentic AI shines when conditions change unexpectedly. If an algorithm controlling inventory levels detects shifting consumer demand, it can adjust orders or distribution schedules without human micromanagement. This adaptability decreases downtime and ensures faster responses to new market shifts.
Proactivity
Proactive AI agents anticipate what might happen next based on patterns, trends, or predictive models. They’re like advanced chess players: always looking a few moves ahead, weighing potential outcomes, and choosing the path that best aligns with organizational goals.
Collaboration with Humans
No AI is a standalone miracle worker. Agentic AI thrives by collaborating with people who bring context, creativity, and values to the table. While the AI handles data-centric tasks, human teams set broader objectives, evaluate ethical implications, and provide nuanced understanding. This synergy allows for a balanced approach: letting AI handle routine tasks while humans maintain strategic control.
Real-World Examples of Agentic AI
Manufacturing and Production
Agentic AI is transforming the manufacturing arena by optimizing resource allocation, predicting machine failures, and even automating entire assembly lines.
Predictive Maintenance
Manufacturers often suffer losses when machines break down unexpectedly. Agentic AI collects sensory data — like vibration analysis, heat readings, and performance metrics — and analyzes potential warning signs. It can then schedule maintenance at the most opportune time, preventing costly disruptions. General Electric, for instance, uses AI-driven predictive maintenance in their aviation and energy sectors, helping them optimize machine usage and preempt failures.
Robotics and Automation
Think about factory robots that do more than simple repetitive tasks. With agentic AI, these robots can reconfigure themselves for various product lines, adapt to new assembly methods, and even collaborate with human workers without direct oversight every step of the way.
Healthcare
In healthcare, agentic AI assists doctors and patients alike, helping to diagnose diseases, design personalized treatment plans, and manage administrative tasks.
Personalized Treatment Plans
Some advanced clinics leverage AI agents to analyze patient data — like blood tests, medical images, and genetic profiles — to devise personalized treatments. Imagine an AI that tailors a chemotherapy regimen to a patient’s unique genetic makeup. This approach, already tested in certain oncology practices, boosts treatment efficacy and reduces adverse reactions.
AI-Powered Diagnostics
Agentic AI can process massive quantities of MRI, X-ray, or CT scan data in real time, spotting subtle anomalies that might slip through human observation. Because these AI systems learn continuously, they become more accurate as they’re exposed to more data, supporting clinical teams in making swift, data-backed decisions.
Supply Chain Management
Agentic AI in supply chain management ensures that the right products get to the right places at the right times — with minimal waste or delay.
Inventory Optimization
An agentic AI system monitors point-of-sale data, warehouse inventories, and production lines. By crunching numbers and spotting patterns, it predicts how much stock is needed — sometimes down to individual store level — thus reducing surplus and freeing up capital.
Route Planning
AI-driven route planning helps logistics companies figure out the most efficient paths for delivery trucks or cargo ships. By analyzing variables like traffic patterns, weather updates, and road conditions, these systems can adapt routes on the go. Companies like UPS have harnessed AI route optimization to save millions of fuel miles and drastically cut delivery times.
Finance and Banking
Agentic AI can help financial institutions with fraud detection, risk assessment, and even investment strategies.
Fraud Detection
Banks and fintech platforms rely on agentic AI to flag suspicious transactions. The system pings anomalies — like sudden overseas withdrawals or unusually large sums — and can lock an account instantly to protect customers. Over time, the AI refines its fraud detection criteria based on new data, making it tougher for fraudsters to slip through.
Automated Portfolio Management
In wealth management, robo-advisors use agentic AI to rebalance portfolios, execute trades, and manage risk thresholds. This isn’t just a static algorithm. If the market takes an unexpected turn, the AI can reorganize allocations on the fly, acting more quickly than a human manager might.
Retail and E-Commerce
Customer Recommendation Engines
Think of Amazon’s “Customers who bought this also bought…” suggestion. Agentic AI can dive deeper, analyzing everything from browsing history to purchasing behavior to personal preferences. It doesn’t just respond to what you last clicked on; it actively predicts future wants and needs, shaping buying patterns.
Dynamic Pricing Strategies
Ever notice how product prices sometimes fluctuate throughout the day? Agentic AI can monitor competitor pricing, customer demand, and inventory levels, adjusting prices instantly. This ensures retailers stay profitable while remaining competitive.
How Agentic AI Collaborates with Human Teams
Enhancing Decision-Making
Agentic AI sifts through mountains of data to offer actionable insights to human experts. It can propose evidence-based strategies, but humans ultimately decide which path aligns with brand identity, culture, or ethics.
Delegating Repetitive Tasks
Data entry, scheduling, routine optimizations — these tasks are prime for AI. By delegating these mundane activities to AI agents, human employees can concentrate on higher-level responsibilities that demand creativity and emotional intelligence.
Human Oversight and Ethics
Despite all the fancy analytics, we must remember that agentic AI is a tool. It’s the human role to ensure the AI’s outputs adhere to ethical guidelines and respect privacy laws. Collaboration means humans stay in the loop, with final oversight on critical issues.
Benefits of Agentic AI
Optimizing Processes
Processes that used to eat up hours — or days — can be completed in a fraction of the time. Assembly lines adjust in real time to production demands, supply chains adapt to shipping anomalies on the fly, and offices run smoother with automated scheduling.
Reducing Costs
Automation typically cuts operational costs by removing excess labor and downtime. Predictive maintenance, for instance, keeps companies from spending big on surprise equipment failures. AI-driven inventory management lowers wasted stock. The result is a leaner, more cost-efficient operation across the board.
Driving Innovation
By freeing human minds from routine tasks, agentic AI promotes a culture of innovation. It empowers teams to brainstorm next-generation products, services, or processes. Plus, these intelligent agents can actually surface new insights from large datasets — hints about customer preferences or market gaps — paving the way for fresh ideas.
Challenges of Implementing Agentic AI
Ethical Considerations
Autonomous systems raise questions about accountability. If an AI agent makes a decision that leads to negative outcomes — like biased loan approvals — who takes responsibility? Clear guidelines and regulatory frameworks are essential to ensure fairness and transparency.
Data Privacy and Security
Agentic AI thrives on data, which can be sensitive. Storing personal information or proprietary business intel opens the door to hacking attempts. Organizations must invest in robust cybersecurity measures and comply with data protection laws to shield both customers and themselves.
Organizational Resistance to Change
Not everyone is ready to embrace new technologies. Employees may worry about job security, while managers might fear losing control. Overcoming this resistance requires transparent communication, offering upskilling programs, and demonstrating tangible benefits.
Best Practices for Successfully Deploying Agentic AI
Setting Clear Goals and KPIs
Define your success metrics from the get-go. Are you looking to reduce inventory waste by 30%? Slash machine downtime in half? Increase fraud detection rates? By having crystal-clear Key Performance Indicators (KPIs), you give your AI agents a target to aim for and your team a metric by which to measure the AI’s success.
Building Cross-Functional Teams
Implementing agentic AI isn’t just an IT project. You’ll need expertise from data science, engineering, operations, finance, and HR. Collaborate across departments to ensure everyone understands the AI’s purpose and functionality.
Iterative Development and Testing
Don’t try to build the ultimate AI system right out of the gate. Launch a pilot program or prototype, gather feedback, refine algorithms, and scale gradually. This iterative process helps you troubleshoot early and fine-tune your approach before large-scale deployment.
The Future of Agentic AI
Emerging Technologies
We’re on the cusp of AI breakthroughs like quantum computing, neuromorphic hardware, and more advanced natural language processing. These technologies will supercharge agentic AI, making it faster, more intuitive, and even more autonomous.
Potential Limitations
AI systems can’t replicate true human empathy or consciousness. They rely on data, which means they’re only as good as the information they consume. If the data is flawed or biased, so will be the AI’s decisions. Moreover, unpredictably large-scale changes — like natural disasters or global economic shifts — can throw off even the most advanced AI models.
Integrating Agentic AI into Existing Workflows
Step-by-Step Implementation
Identify the Right Process: Pinpoint repetitive or data-intensive tasks that could benefit from automation.
Select Suitable Tools: Explore AI platforms and frameworks that suit your industry.
Pilot Program: Start small by running a test scenario and measuring performance.
Evaluate and Optimize: Adjust algorithms based on feedback, then roll out the AI across other departments or processes.
Tools and Resources
Cloud Platforms: AWS, Azure, or Google Cloud for scalable computing and machine learning services.
Open-Source Frameworks: TensorFlow, PyTorch, and other libraries for building and training AI models.
Enterprise Solutions: Off-the-shelf platforms like IBM Watson or Microsoft’s Cognitive Services can speed up deployment.
Case Studies of Agentic AI
Amazon
Warehouse Automation: Amazon’s Kiva robots transport products around fulfillment centers autonomously, optimizing routes in real time.
AI-Driven Demand Forecasting: The company uses machine learning to predict order volumes, ensuring that items are stocked in the right quantities at the right locations.
Tesla
Self-Driving Technology: Tesla’s Autopilot employs agentic AI to make split-second decisions on the road — like changing lanes, adjusting speed, or braking for obstacles.
Factory Automation: Tesla’s Gigafactories integrate robotics and AI-driven systems to optimize battery production and streamline car assembly, adapting to changes in production demands.
IBM
Watson in Healthcare: IBM’s Watson can analyze vast medical datasets to suggest treatment options for cancer. It adapts as new research emerges, becoming more accurate over time.
AI in Supply Chain: IBM leverages agentic AI tools to help companies manage everything from supplier relations to logistics, adapting to new data inputs instantly.
The Human Element in AI
Continuous Learning and Upskilling
As AI takes over routine tasks, human workers have the opportunity to shift to more creative, strategic, and interpersonal roles. Upskilling programs help employees transition. Rather than being replaced, workers learn to coexist with AI, focusing on complex problem-solving and emotional intelligence.
Maintaining Control and Accountability
Even the most advanced agentic AI needs human oversight, especially in critical industries like healthcare or finance. Humans set the objectives, handle exceptions, and ensure that decisions align with ethical and societal norms. While AI might crunch data, we retain the responsibility for final decisions.
Measuring the Impact of Agentic AI
Performance Metrics
Measure outcomes like reduced operational costs, increased quality, and heightened customer satisfaction. Keep track of both short-term milestones (like faster turnarounds on projects) and long-term objectives (like market expansion or stronger brand loyalty).
Employee Engagement
Don’t overlook how agentic AI affects your workforce. Surveys, interviews, or focus groups can reveal whether employees feel empowered by AI or threatened by it. Positive employee engagement often correlates with more successful AI rollouts.
Conclusion
Agentic AI is not just a fleeting buzzword; it’s reshaping how we do business. From robotics on the assembly line to dynamic pricing in retail, these autonomous systems are weaving their way into countless industries. The key to getting the most out of this technology lies in balancing AI-driven autonomy with the human spark — our creativity, empathy, and big-picture thinking. By pairing agentic AI with well-trained teams and a clear strategy, organizations can revolutionize processes, slash unnecessary costs, and spark innovative ideas that set them apart in an ultra-competitive market.
FAQs
How does agentic AI differ from traditional automation?
Traditional automation follows predefined rules without deviation, while agentic AI can adapt to real-time changes, learn from new data, and make decisions autonomously.
Do I need a large budget to implement agentic AI in my business?
The initial investments vary, but cloud services and open-source tools have made agentic AI more accessible. Smaller businesses can start with pilot projects and scale gradually.
Will agentic AI replace human workers?
Agentic AI aims to augment human roles rather than eliminate them. It takes over repetitive tasks, freeing humans to focus on strategic, creative, and interpersonal responsibilities.
What are the biggest security concerns with agentic AI?
Data breaches and unauthorized access to AI systems are major issues. It’s crucial to implement robust cybersecurity measures and comply with data privacy laws to mitigate these risks.
How do I ensure the AI remains unbiased?
Regular audits, transparent data collection, and diverse development teams are key. If data is skewed, the AI’s decisions will be too. Ongoing monitoring and corrections help maintain fairness.
Comments